Evolution Trend of Network Intelligence
Since its birth, telecom network has undergone several stages such as analog, digital, Internet, and mobile Internet communications. Each stage has greatly improved social productivity and the development of new industries. The emergence of 5G, one of key technologies in the Fourth Industrial Revolution, will lead to great changes in social production and human life. Because of its digital, cloud-based and micro-service features, 5G network will give rise to a large number of new services for vertical industries, so it will also have to face the differentiated customer requirements and improved standards brought about by the new services. This will make network operation and maintenance (O&M) increasingly complicated, and intelligence is the key to solving these problems.
130 representatives from 33 companies, led by Deutsche Telekom, held a seminar on future network and service management in December 2016, which was a prelude to network automation. At that time, the network adopted the traditional network management (NM) and manual management mode, and its O&M mode was also passive to solve problems. With the applications of SDN, the network has become programmable, and NM no longer merely plays a management role. With the help of the controller, the network can perform some active O&M operations such as network simulation and early warning. 5G networks have been gradually deployed across the world since 2019, and the world has ushered in the era of network intelligence. With the development of big data collection and analysis as well as the introduction of AI, the network can intelligently process all kinds of network information in real time, such as service provisioning, intelligent fault location, and automatic network optimization. The network will evolve to an autonomous network in the future. As the intention concept is introduced, the network will have the same "self-awareness" as the human brain. Based on the rules set by the network manager, the network implements closed-loop self management of requirements, policies, decisions, implementation, maintenance and optimization, which can completely liberate the manpower.
Knowledge: Core of Network Intelligence
Just as human wisdom comes from production, living and learning activities, network intelligence is also gradually formed after repeated training based on massive data. The current network adopts SDN architecture, collects various network data through Telemetry, and uses AI algorithms to learn, so it has a certain degree of intelligence. However, as the requirements for intelligence become more and more specific, the amount of network data that needs to be collected increases exponentially. When actual computing resources are limited, if data is processed only through the AI algorithms, it will be difficult for the network to provide the intelligence of self management and control. To get out of this difficulty, it is necessary to make use of knowledge, including the knowledge extracted from human experience and discovered through the AI technology. The organic cooperation of knowledge and AI can lower the requirements for data processing and computing resources, and improve the efficiency and capability of network intelligence.
The difference between human beings and other living creatures is that they can constantly form systematic knowledge and use it to transform the world. In general, knowledge is systematic and verifiable information that can be formalized and normalized. At present, the AI technology used in telecom networks is to solve a problem in a scenario by using an AI algorithm. There is no accumulation of knowledge points in the system, let alone the establishment of a systematic knowledge set, so it is impossible to realize knowledge-based reasoning, decision-making and high-level intelligence like human beings. Therefore, in terms of intelligence development, having some AI algorithms and capabilities is not equal to intelligence. The core of intelligence is to have systematic knowledge and the ability to effectively use and automatically update the knowledge.
Knowledge and AI Complement Each Other in Network Intelligence
In network intelligence, knowledge and AI complement each other, just like a person's left hand and right hand. AI helps to find the intrinsic chain of relationships hidden in isolated information, which accelerates knowledge acquisition and system establishment, while knowledge can help to remove invalid information among massive raw data during AI learning and improve the speed of AI learning and training.
AI Enhances Knowledge Acquisition
Before AI, knowledge acquisition is achieved by standardizing expert experience and formalizing network technologies. As expert experience is different, incomplete and not updated in time, it cannot meet the actual network requirements. The introduction of AI makes knowledge acquisition more active and effective, and forms a complete, standard, and available set of systematic network knowledge through the knowledge management. AI algorithms for knowledge acquisition include clustering analysis, time series, maximum entropy model, decision tree, isolation forest, vector machine, and hidden Markov model. Moreover, new algorithms continue to emerge. The knowledge acquired by AI can extract entities, relations, attributes and rules, such as abnormal features, correlations, fault types, and number of faults. Such knowledge can be integrated with the knowledge provided by human experts such as network architecture, resource data, service models, O&M modes, and processing procedures to form associated, scenario-based, and systematic network knowledge sets. The combination of human intelligence and AI ensures effective knowledge generation and update. Take alarm processing as an example, after the alarms are suppressed according to the experience of experts in operation and maintenance, the number of alarms is reduced by 50%. Through the AI-based rule mining, the number of alarms is further reduced to the initial 10%.
Knowledge-Based Data Selection Improves AI Efficiency
Data is the basis of network intelligence. Due to the wide coverage and complexity of network data, the collection, processing, and storage of massive data is a great challenge. With the improvement of network intelligence and the richness of network knowledge, precise data can be selected through knowledge management and decision making, thus improving the AI processing efficiency. In the 5G era, network experience can be achieved in seconds or even milliseconds. But if all network information is reported and processed in this order of magnitude, the existing network cannot support it with its current communication capability, computing power and storage capacity. However, using certain knowledge rules, the system can determine what data can be collected in seconds for a period of time as required, and other data can be collected in minutes or even hours. The optimal AI algorithm can also be matched to achieve efficient and intelligent network experience based on precise data selection.
Reasoning Enables Knowledge Discovery
The AI technology plays an important role not only in knowledge acquisition but also in knowledge update and discovery, which is achieved through knowledge reasoning. AI algorithms for knowledge reasoning include reasoning based on rules (such as first order logic and deductive reasoning), reasoning based on statistical learning (such as machine learning, Bayesian reasoning, and Markov logic network), reasoning based on graphs (such as semantic reasoning, structure-based reasoning, and reasoning based on knowledge graph representation and learning), and integrated reasoning that combines knowledge graphs and deep learning. The AI-assisted knowledge reasoning helps to discover new knowledge or incorrect knowledge more quickly and supports decision-making to dynamically update and maintain knowledge. Taking the fault analysis and location function as an example, the knowledge graph is completed through AI or expert experience. In addition, the AI-assisted knowledge reasoning can also help to build a fault transmission chain and finally identify the root cause of faults. It can be seen that AI simplifies and accelerates the discovery of network knowledge, and improves the self-development capability of network intelligence. Therefore, the network knowledge without AI is incomplete, and the AI without network knowledge is unreal.
Development Route of Knowledge and AI in Network Intelligence
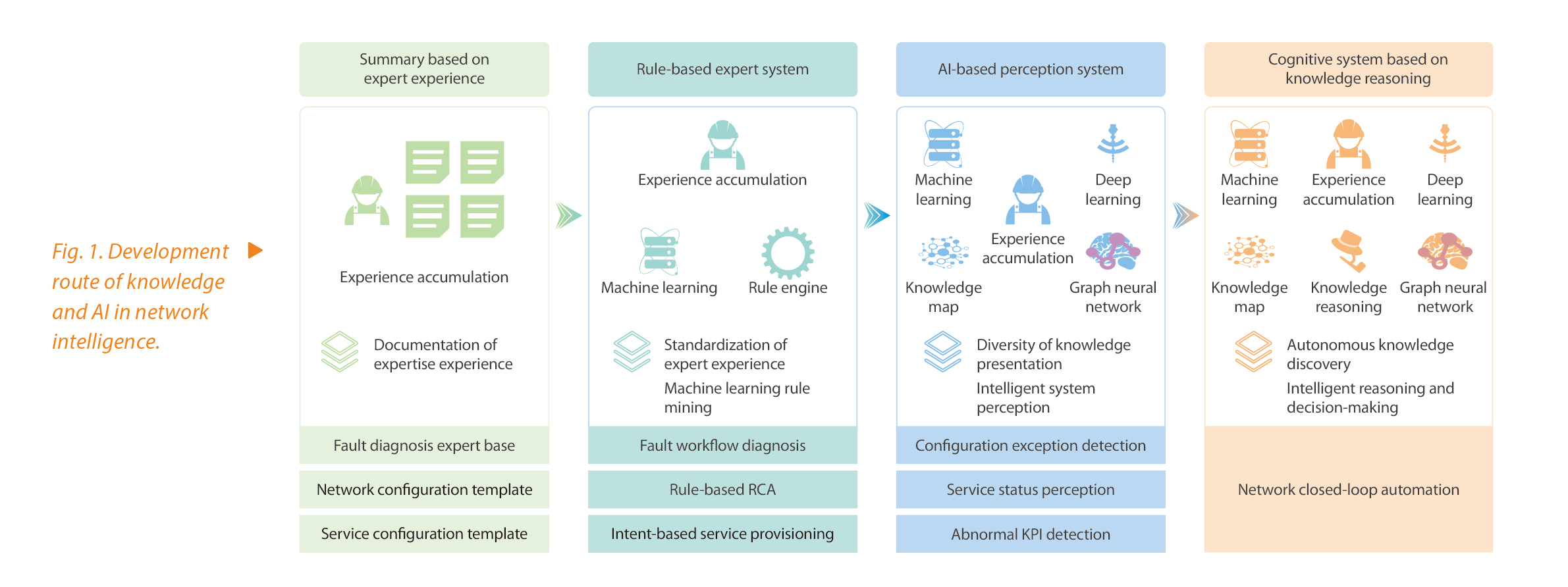
Knowledge and AI play a major role in the development of telecom network intelligence, and their development route can be divided into four stages (Fig. 1). The first stage is a summary based on expert experience, where the knowledge based on human expert experience is presented as knowledge questions and answers, or presented as fault diagnosis expert base, network configuration template, and service configuration template in the system. In this stage, AI is introduced, the content of knowledge is fixed, and its expression is not planned or systematic, so the role of knowledge is quite limited. With the preliminary introduction of AI, the system evolves to a rule-based expert system. In this stage, the experience of human experts is regulated, and new rules are mined through machine learning to form a series of standardized knowledge. The rule engine can be used to automatically execute some functions, such as fault workflow diagnosis and rule-based RCA. With the further development of AI, the system evolves to the AI-based perception system. In this stage, multiple AI technologies such as graph neural network, deep learning, and knowledge graph, are used to acquire and present knowledge in a different way, and gradually form a systematic knowledge set to achieve intelligent network perception. The application instances available include intelligent configuration exception detection, service status perception, and abnormal KPI detection. With the in-depth understanding of knowledge and AI technologies, a cognitive system based on knowledge reasoning will be formed finally. In this stage, a complete, dynamic and systematic knowledge set will be established, with the intent-based network as the core to realize autonomous knowledge discovery as well as intelligent reasoning and decision-making, thus enabling a closed-loop network and service intelligence during their whole lifecycle.
As 5G networks develop rapidly, it is believed that practice and demands are the strongest impetus for technological development, and the closer combination of knowledge building and AI technology may generate new systems that exceed our expectations. With great technical strength and rich experience in the telecom field, ZTE will actively work with partners to lead the future development of network intelligence and carry out its great mission of being a road builder of digital economy.